Bridging the Generative AI Talent Gap: Empowering Prompt Engineers for AI Adoption
Updated: Feb 27, 2024
Building a Future-Ready Workforce for Generative AI Adoption
As GenAI gains rapid adoption in organizations, the unique nature of its usage and implementation sets it apart from traditional machine learning approaches. With the rise of Large Language Models (LLMs), particularly in the context of prompt engineering, it promises quick and cost-effective solutions for companies. However, this shift has also exposed a significant talent gap in the field of data science, specifically in prompt engineering roles.
This talent shortage necessitates proactive steps by companies to develop pathways for experts and transform their operations to harness the power of Generative AI for accelerating business value.
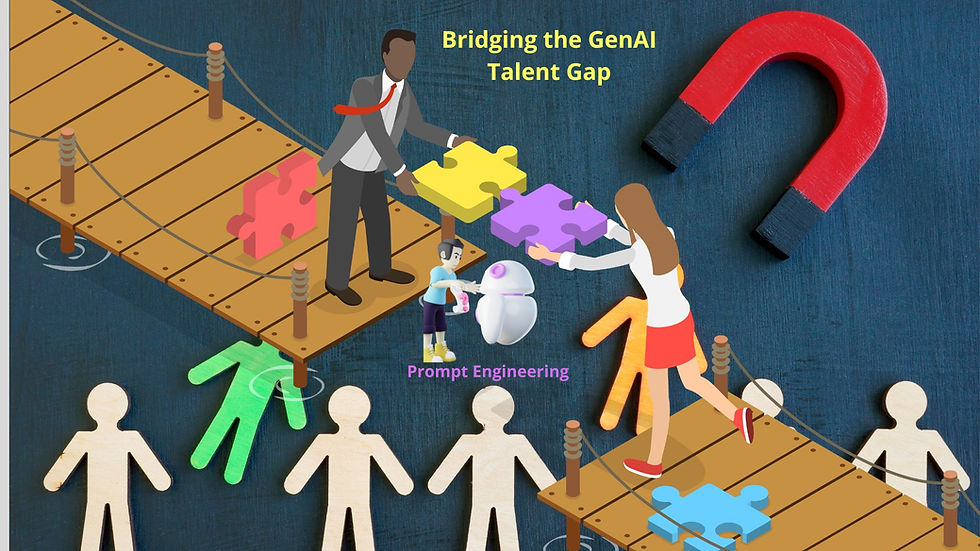
Bridging the Talent Gap in Prompt Engineering
The journey to address this talent gap begins with elevating communication and awareness about data science and machine learning across the organization. It is essential for leaders and decision-makers to gain a clear understanding of how data scientists leverage Generative AI to create value. Workshops and discussions should encourage questioning of existing Key Performance Indicators (KPIs), data sources, and metrics.
The Role of a Prompt Engineer
A prompt engineer plays a pivotal role in interacting with AI models, providing business knowledge to enhance the model's accuracy and effectiveness. They serve as collaborators between machines and humans. To excel in this role, prompt engineers must possess a unique blend of hard skills and business acumen.
Hard Skills:
· Understanding of how Large Language Models (LLMs) work.
· Proficiency in APIs and programming languages like Python.
· Skills in Natural Language Processing (NLP) and ML performance evaluation metrics.
Business Skills: Deeper domain knowledge in specific business areas compared to traditional data scientists.
The Prompt Engineer as a Citizen Data Scientist
In many cases, a prompt engineer can also be a citizen data scientist, positioned between analytics consumers on the business side and data scientists. Organizations should develop a strategic upskilling plan that spans three stages to bridge the gap between the technical expertise of data scientists and the domain knowledge of business users.
1. Build a Culture of Collaboration with Diverse Talents
Promote collaboration between data scientists and business leaders to ensure a shared understanding of the value prompt engineers bring. Encourage workshops and discussions to elevate discussions around business needs, data science and machine learning.
Foster interconnected communities of data scientists, citizen data scientists, and other stakeholders in the Generative AI pipeline. Facilitate collaboration, mentorship, and strategic investments in software tools to support their growth.
2. Continuous Learning
Support the ongoing development of expert prompt engineers by providing opportunities for independent learning and growth in emerging AI domains. Establish centralized educational resources to enhance data science and GenAI awareness. Highlight both internal and external use cases and success stories to inspire and educate.
Identify potential prompt engineering candidates by evaluating their skills and aspirations. Look for individuals with backgrounds in fields such as mathematics, computer science, and specific industry knowledge.
3. Upskilling Roadmaps
Develop structured upskilling programs tailored to both Citizen Data Scientist (CDS) candidates and analytics consumers. These programs should align with individuals' backgrounds, aspirations, and skillsets.
Training Methods: Define career paths and provide formal training through courses, boot camps, or tool-specific programs. Leverage resources like Massive Open Online Courses (MOOCs) for learning.
Proof of Concept (POC): Encourage experimentation in sandbox environments, guided by experienced trainers.
Enterprise Solutions: Following successful Proof of Concept (POC) projects, prompt engineers gain valuable expertise, enabling them to undertake the development of larger and more advanced models.
To foster a generative AI culture and ensure rapid adoption of this transformative technology, companies must create robust upskilling roadmaps. By following these structured programs, encouraging collaboration, and prioritizing continuous learning, organizations can cultivate a data-savvy workforce ready to bridge the talent gap and harness the vast potential of Generative AI for innovation and growth.
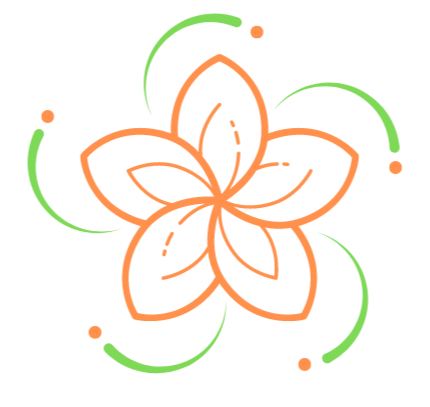
May you grow to your fullest in your data science & AI!
Subscribe Grow to Your Fullest and get your Free Download
*** Please DOWLOAD the FREE document, Find your signature vision questionnaires so you use it to help you find your life vision and mission.
>> Connected on Facebook
コメント